Types of AI: From Narrow to Artificial General Intelligence (AGI)
- The Tech Platform
- Mar 18, 2024
- 6 min read
Artificial intelligence (AI) is intelligence demonstrated by machines, in contrast to the natural intelligence displayed by humans and animals. AI systems are programmed to learn and adapt, allowing them to perform tasks that require human intelligence.
AI is rapidly transforming our world. From facial recognition software to self-driving cars, AI is making its way into an increasing number of applications. This trend is only expected to continue in the future, as AI research continues to advance.

Not all AI is created equal. There are different types of AI, each with its strengths and weaknesses. In this section, we will explore some of the major types of AI, focusing on the distinction between Artificial Narrow Intelligence (ANI) and Artificial General Intelligence (AGI).
Types of AI
There are two types of AI:
Artificial Narrow Intelligence (ANI)
Artificial General Intelligence (AGI)
Artificial Narrow Intelligence (ANI)
Artificial Narrow Intelligence (ANI), also known as weak AI, is a type of AI that excels at performing specific, well-defined tasks. Unlike a human who can learn and adapt to new situations, ANI is limited to the capabilities it has been programmed for.
Characteristics:
Task-Specific: ANI is designed to handle a single task or a narrow range of similar tasks. They are trained on vast amounts of data specific to their designated purpose. For example, a facial recognition system is exceptional at identifying faces within an image, but wouldn't be able to write a poem.
Limited Abilities: ANI lacks the general intelligence and adaptability of humans. They cannot reason outside the parameters they are trained on and struggle with situations requiring broad problem-solving skills.
Examples of ANI Applications:
Facial Recognition Software: Used for security purposes, photo tagging, and even unlocking smartphones.
Spam Filters: Automatically identify and remove unwanted emails from your inbox.
Chess-Playing Programs: Can analyze vast quantities of chess games and strategies to defeat even grandmasters.
Recommendation Systems: Suggest products or content you might be interested in based on your past behavior (e.g., online shopping recommendations).
Self-Driving Cars: Utilize computer vision and sensor data to navigate roads within a predefined set of conditions.
Strengths of ANI:
High Accuracy: ANI excels at performing repetitive tasks with high accuracy.
Efficiency: ANI can process information and complete tasks much faster than humans.
Cost-Effective: Once developed, ANI systems can be relatively inexpensive to implement and maintain.
Weaknesses of ANI:
Limited Scope: ANI cannot adapt to new situations or perform tasks outside their specific programming.
Lack of Reasoning: ANI cannot reason or make judgments based on incomplete information.
Vulnerability to Bias: ANI systems can inherit biases from the data they are trained on.
Learning Techniques of ANI:
Machine Learning: ANI systems often rely on machine learning algorithms to learn from data. These algorithms identify patterns and relationships within the data, allowing the ANI to improve its performance over time. For instance, a spam filter might learn to identify spam emails based on keywords, sender addresses, and other factors.
Deep Learning: A subfield of machine learning, deep learning utilizes artificial neural networks loosely inspired by the human brain. These networks can process complex data sets and perform tasks like image recognition with exceptional accuracy. Facial recognition software often leverages deep learning for this purpose.
Beyond Simple Tasks:
While typically confined to specific tasks, ANI can exhibit impressive capabilities within its domain.
Chess Mastery: ANI chess programs like AlphaZero can not only defeat human champions but also discover novel and creative strategies unimaginable to human players.
Medical Diagnosis: ANI systems can analyze medical scans and patient data to assist doctors in diagnosing diseases with higher accuracy.
The Future of ANI:
Continuous Improvement: As machine learning and data collection techniques advance, ANI systems will likely become even more sophisticated and capable of handling more complex tasks.
Human-AI Collaboration: The future may see a rise in human-AI collaboration, where ANI takes care of routine tasks while humans focus on higher-level decision-making and problem-solving.
Ethical Considerations:
Bias in AI: As mentioned earlier, ANI systems can inherit biases from the data they are trained on. This raises ethical concerns, such as biased hiring algorithms or facial recognition systems disproportionately misidentifying people of color. Mitigating bias in AI development is crucial.
Job Displacement: The increasing automation of tasks by ANI could lead to job displacement in certain sectors. This necessitates preparing the workforce for new opportunities created by AI.
Artificial General Intelligence (AGI)
Artificial General Intelligence (AGI), also known as strong AI, represents the holy grail of AI research. It expects machines to possess human-level intelligence, capable of understanding and reasoning across topics and situations. Unlike ANI's laser focus on specific tasks, AGI would exhibit broad problem-solving skills and adaptability, akin to the human mind.
Characteristics of AGI:
Human-Level Intelligence: AGI would possess cognitive abilities comparable to humans, including learning, reasoning, planning, and problem-solving in complex and open-ended situations.
General Intelligence: AGI wouldn't be restricted to a single domain. It could apply its intelligence to various tasks, similar to how humans can learn a new skill or solve a novel problem.
The term "strong AI" is often synonymous with AGI. It implies an intelligence that rivals or surpasses human capabilities, not just in performance but also in its ability to understand and be conscious of its surroundings.
Challenges to AGI:
Developing AGI remains a significant hurdle in AI research. Here are some key challenges:
Replicating Human Reasoning: Human reasoning involves a complex interplay of logic, intuition, and experience. Recreating this ability in machines is a major challenge.
The Mystery of Consciousness: Whether or not machines can achieve true consciousness, a state of self-awareness and sentience, remains an open question. Without understanding consciousness, replicating it in AI becomes even more difficult.
Benefits of AGI:
The potential benefits of AGI are vast. Imagine AI systems that can revolutionize scientific discovery, tackle global challenges like climate change, or even provide companionship and care for humans. However, concerns also exist:
Existential Risk: Some experts warn that superintelligent AGI could pose an existential threat if its goals diverge from humanity.
Job Displacement on a Grand Scale: If AGI can perform most tasks currently done by humans, widespread unemployment could become a major issue.
Theoretical Approaches to AGI:
There's no single agreed-upon path to achieving AGI. Researchers explore various approaches:
Symbolic AI: This approach manipulates symbols and rules to represent knowledge and reasoning. While successful in specific domains, it struggles with the complexity of real-world situations.
Artificial Neural Networks: Inspired by the human brain, these networks process information through interconnected nodes. Deep learning, a subfield of this approach, has shown promising results in tasks like image and speech recognition, but replicating general human intelligence.
Artificial Consciousness Research: Some researchers believe true AGI requires achieving artificial consciousness. This field explores the nature of consciousness and how to instill it in machines, posing significant philosophical and ethical questions.
The technological singularity is a hypothetical future moment when AGI surpasses human intelligence, potentially leading to rapid and unpredictable technological change. Some experts believe it's inevitable and could happen within this century, while others consider it a distant possibility or even a misconception.
Given the potential risks of AGI, AI safety research is crucial. This field focuses on developing safe and beneficial AGI, ensuring its goals are aligned with human values. Strategies include developing robust safety protocols, building ethical considerations during AI development, and fostering international collaboration on AI governance.
Even with the advent of AGI, human intelligence is unlikely to become obsolete. The future might see a symbiotic relationship, where humans and AGI collaborate, leveraging each other's strengths. Humans could provide guidance and ethical oversight, while AGI tackles complex problems or automates mundane tasks.
The quest for AGI is both a scientific and philosophical endeavor. While the challenges are significant, the potential benefits are equally vast. By approaching AGI development responsibly and focusing on human well-being, we can harness its power for a brighter future.
Difference Between Types of AI: ANI vs AGI
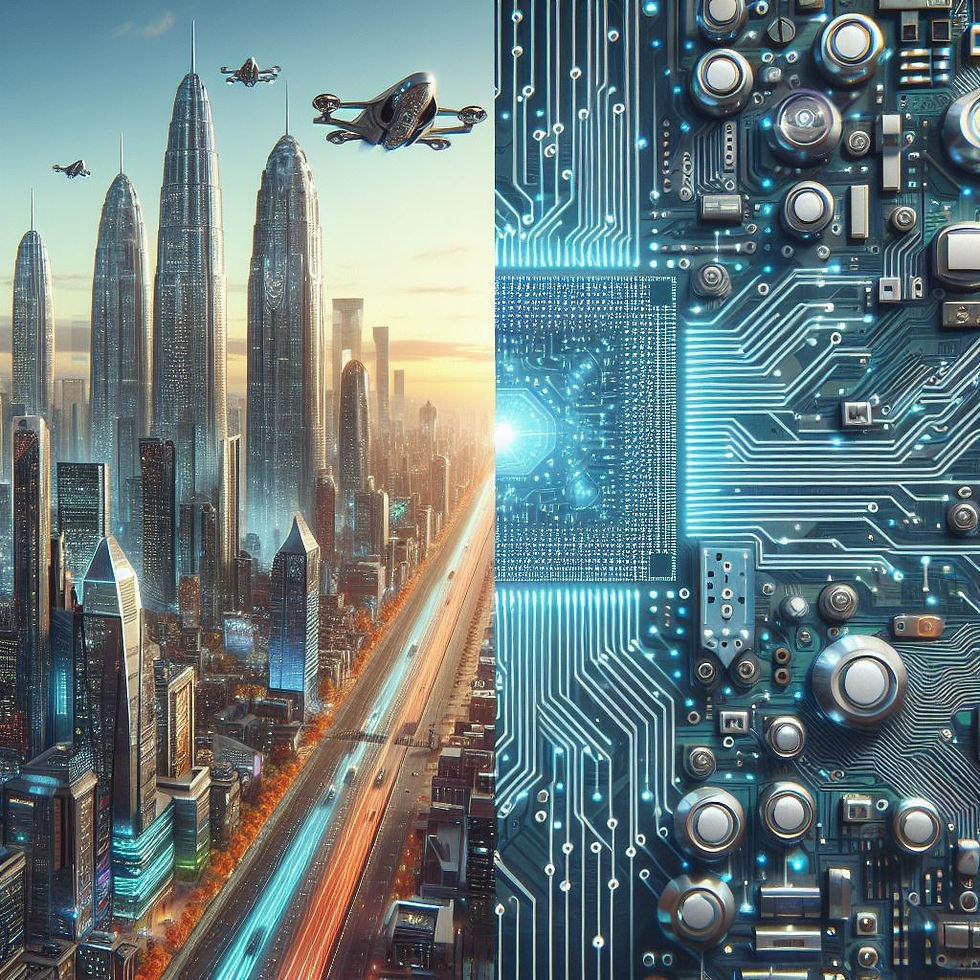
Factors | Artificial Narrow Intelligence | Artificial General Intelligence |
Learning and Adaptability | Relies on pre-programmed data and algorithms for specific tasks. Limited ability to adapt to new situations or learn outside its training set. | learn and adapt continuously, similar to how humans acquire new knowledge and adjust their approach based on experiences. |
Data Dependency | Heavily dependent on vast amounts of labeled data for training. Performance can be hindered by the quality and quantity of data available. | May require less reliance on pre-labeled data, potentially possessing the ability to learn from the world around it and generalize its knowledge. |
Level of complexity | ANI systems are typically built on well-defined rules and algorithms, making them easier to develop and understand. | Replicating the human mind's complexity, encompassing aspects like consciousness, emotion, and common sense, is a significant challenge for AGI development. |
Creativity and Innovation | ANI excels at following established procedures and replicating learned patterns. While some ANI systems exhibit impressive performance within their domain, they generally lack true creativity or the ability to innovate. | AGI, if achieved, could potentially exhibit genuine creativity and come up with novel solutions or ideas not explicitly programmed into it. This ability could lead to breakthroughs in various fields. |
Conclusion
Artificial Narrow Intelligence (ANI) and Artificial General Intelligence (AGI) represent two distinct types of AI. Here's a recap of their key differences:
Scope: ANI excels at specific tasks, while AGI aspires to human-level intelligence across various domains.
Learning: ANI relies on pre-programmed data, whereas AGI envisions continuous learning and adaptation.
Explainability: ANI's decision-making process is often opaque, while AGI ideally would be transparent in its reasoning.
Impact: ANI drives existing applications, whereas AGI could revolutionize society, but also raises ethical concerns.
Development: ANI is well-established, with many applications in use today. AGI remains theoretical, with a distant, uncertain timeline.
AI research continues to push boundaries. Advancements in machine learning and artificial neural networks hold promise for the future of AI. However, significant challenges remain in areas like replicating human reasoning and consciousness.
Whether or not we will achieve AGI is a topic of debate. Some experts believe it's inevitable, while others consider it a distant possibility or even a misconception. Regardless, focusing on responsible AI development, mitigating risks, and fostering human-AI collaboration will be crucial as we navigate the future of AI.
Comments