In today’s competitive market, businesses are under tremendous pressure to develop innovative products and bring them to market quickly. However, not all products are successful, and failure can be costly, both in terms of resources and reputation. That's why it is important to use data mining techniques to predict whether a product will crash and burn before it even hits the shelves.
Data mining is a process of discovering patterns and knowledge from large datasets. It involves the use of various techniques such as clustering, classification, and association rule mining to analyze data and extract valuable insights. By using data mining, businesses can analyze their past performance, customer behavior, market trends, and other relevant data to predict the success or failure of their products.
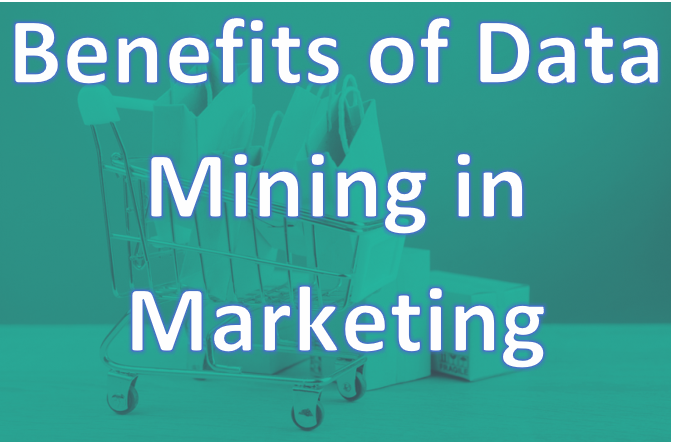
TOP BENEFITS OF DATA MINING IN MARKETING
Here are some of the top benefits of data mining in marketing:
Basket Analysis: What is it? Uncovering which products or services are often purchased together. How does it use data mining? Association rule learning.
Product Recommendation: What is it? Tailoring product suggestions to individual users based on data. How does it use data mining? Association rule learning, along with techniques like collaborative filtering and content-based filtering.
Customer Segmentation: What is it? Subgrouping customers or clients into subsets based on common characteristics and habits. How does it use data mining? Cluster analysis.
Customer Lifetime Value: What is it? Quantifying how much money a customer is likely to generate for a company. How does it use data mining? Decision trees and boosting.
Churn Prediction: What is it? Quantifying the likelihood a customer will stop doing business with a company. How does it use data mining? Classification and regression.
BASKET ANALYSIS
Basket analysis is a technique that is used to uncover which products or services are often purchased together. By using association rule learning, businesses can identify patterns in customer behavior and use this information to create targeted marketing campaigns. For example, a business might use basket analysis to determine that customers who purchase coffee beans also frequently purchase a particular brand of coffee grinder, and then use this information to create a targeted promotion that offers a discount on both products when purchased together.
Example:
A grocery store chain used basket analysis to uncover patterns in customer behavior and optimize its product offerings. By analyzing customer purchases, the company discovered that customers who purchased beer on weekends were also more likely to purchase chips and salsa. Armed with this information, the grocery store created a targeted promotion that offered discounts on beer and chips/salsa when purchased together. As a result, sales of both products increased significantly.
PRODUCT RECOMMENDATION
Product recommendation is a technique that involves tailoring product suggestions to individual users based on data. By using association rule learning, along with techniques like collaborative filtering and content-based filtering, businesses can create personalized product recommendations that are more likely to result in a sale. For example, an e-commerce website might use collaborative filtering to suggest products to customers based on their past purchase history or browsing behavior.
Example:
An online retailer used product recommendations to personalize their customers' shopping experiences. By analyzing customer purchase history and browsing behavior, the company was able to suggest products that were more likely to be of interest to each individual customer. This resulted in increased customer engagement, higher conversion rates, and higher average order values.
CUSTOMER LIFETIME VALUE
Customer segmentation is a technique that involves sub-grouping customers or clients into subsets based on common characteristics and habits. By using cluster analysis, businesses can create targeted marketing campaigns that are more likely to resonate with specific customer groups. For example, a business might use customer segmentation to create a targeted promotion for customers who have purchased a specific product in the past.
Example:
A car manufacturer used customer segmentation to create targeted marketing campaigns for different customer segments. By analyzing customer data, the company identified several key customer segments, including environmentally conscious consumers, families, and luxury car buyers. The company then created tailored marketing campaigns for each segment, resulting in increased sales and higher customer satisfaction.
CUSTOMER SEGMENTATION
Customer lifetime value is a metric that quantifies how much money a customer is likely to generate for a company over the course of their relationship. By using decision trees and boosting, businesses can predict customer behavior and use this information to make informed decisions about marketing strategies and product development. For example, a business might use customer lifetime value to determine whether it makes sense to invest in a loyalty program that rewards long-term customers.
Example:
A subscription-based service used customer lifetime value to identify and retain their most valuable customers. By analyzing customer data, the company was able to identify customers who were likely to generate the most revenue over the course of their subscriptions. The company then created targeted promotions and offers to incentivize these customers to continue their subscriptions. As a result, the company saw increased customer retention and higher revenue.
CHURN PREDICTION
Churn prediction is a technique that involves quantifying the likelihood that a customer will stop doing business with a company. By using classification and regression, businesses can predict customer behavior and take steps to prevent churn. For example, a business might use churn prediction to identify customers who are at high risk of churning and offer them a discount or other incentive to encourage them to stay.
Example:
A telecommunications company used churn prediction to identify customers who were at risk of leaving their service. By analyzing customer data, the company was able to identify several key factors that were associated with high churn rates, such as poor signal quality and long wait times for customer service. The company then took steps to address these issues, resulting in decreased churn rates and higher customer satisfaction.
Conclusion
In conclusion, data mining can be a powerful tool for businesses looking to gain insights into customer behavior, market trends, and other relevant data. By using techniques like basket analysis, product recommendation, customer segmentation, customer lifetime value, and churn prediction, businesses can make informed decisions about product development, marketing strategies, and other important business decisions.
Comments